In the modern business world, data is king—and A/B testing unlocks critical data resources, especially for online businesses.
While many companies conduct A/B testing, not all use objective-oriented A/B testing strategies to maximize efficiency. The right approach to A/B testing can help business leaders design highly effective experiments, produce reliable results, and access troves of valuable data.
We recommend these best practices when designing your A/B testing roadmap:
- Identify your company’s business objectives.
- Determine a hypothesis for each experiment.
- Understand the primary and secondary objectives of each test.
- Set a strict experimentation timeline.
- Limit the number of variations per test.
Ready to learn more? Here’s a breakdown of our top A/B testing strategy best practices.
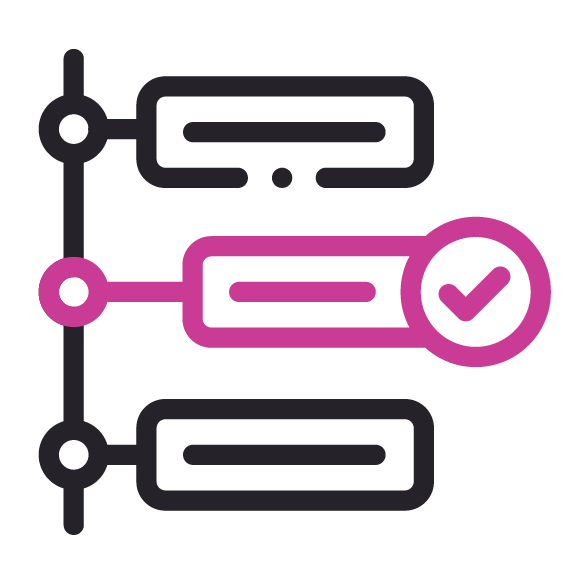
Outline Your Top Goals and KPIs in a Goal Tree
To set up your company for successful A/B testing, start by evaluating your business goals, identifying your primary business objective, and outlining an optimization strategy with KPIs that lead up to your primary goal, or the “north star.” Here at BlastX Consulting, we use a goal tree to create this outline.
Let’s say we’re outlining goals for an ecommerce company — we’ll call it Bizness Inc. — whose primary objective is generating revenue. Generating revenue would be the north star and sit at the top of the goal tree. The second level of the tree would comprise the KPIs contributing to revenue generation; these might include conversion rate, average order value, and add-to-cart rate.
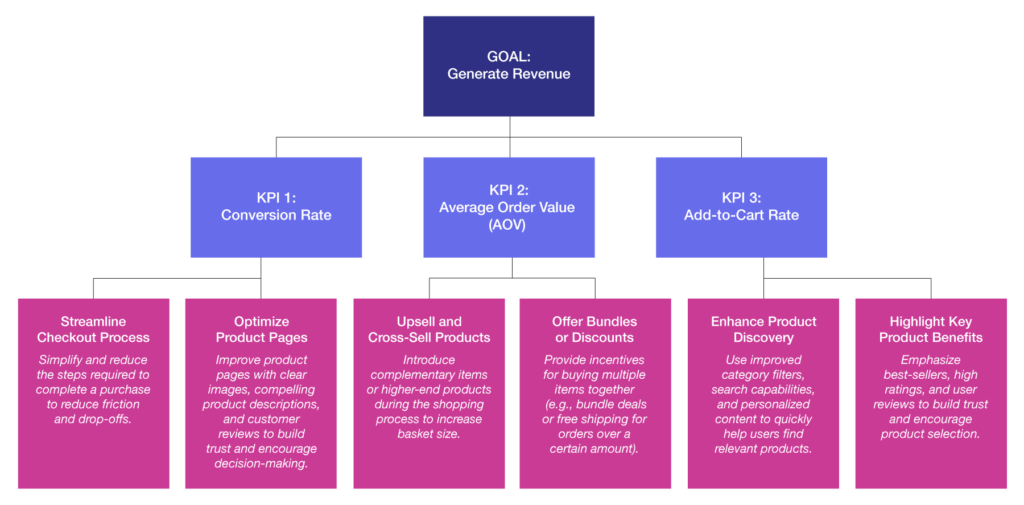
When we run A/B tests, we aim to improve our KPIs — but how? Outlining optimization strategies is the next step. We recommend coming up with three to six optimization strategies to help your company achieve its primary goal. You can use A/B testing to determine the best way to implement those strategies.
Take our hypothetical Bizness Inc., for example: Streamlining the purchase path (optimization strategy) could help increase the conversion rate (KPI), which would in turn help generate revenue (north star). Bizness might run an A/B test to see whether adding ApplePay for faster checkout would effectively optimize its purchase path.
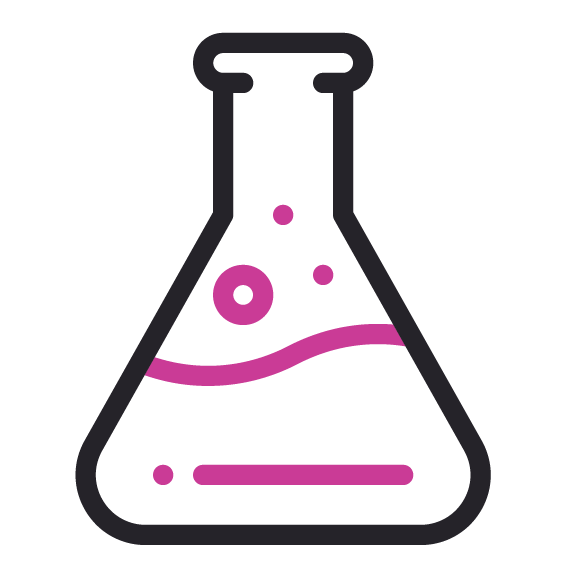
Establish a Clear Hypothesis for Each Test
A/B testing is part creativity and part science. The creative part consists of brainstorming exciting new tests; the science part involves the experiment itself. And every experiment needs a hypothesis.
The hypothesis format for an A/B test typically outlines the problem, solution, and result. Let’s return to our previous example: Bizness Inc. wants to use A/B testing to determine whether implementing Apple Pay would optimize the purchase path and increase its conversion rate. The hypothesis for this experiment might look like:
Implementing Apple Pay on Bizness Inc.’s website will increase conversion rates by decreasing additional checkout steps for customers and optimizing the purchase path.
- Problem: Our customers are dropping off the payment page because it takes too long for them to enter their card number and address.
- Solution: Implementing Apply Pay will optimize the checkout process by allowing customers to skip entering their payment information.
- Results: Adding a new payment method will increase the conversion rate among users who have started the checkout process.
Knowing the pain points along the customer journey helps business leaders outline and define the problem so they can start looking for a solution.
The key to a well-formulated hypothesis is understanding the data and reasoning behind the test. Knowing the pain points along the customer journey helps business leaders outline and define the problem so they can start looking for a solution. Many articles show that Apple Pay increases the conversion rate among customers in a hurry. In the above example, Bizness Inc.’s hypothesis is rooted in evidence from both internal data and use cases from other companies.
The hypothesis is a starting point created from an identified problem. And if you run an A/B test and the results aren’t what you expected, you’ve identified a new problem to start testing.
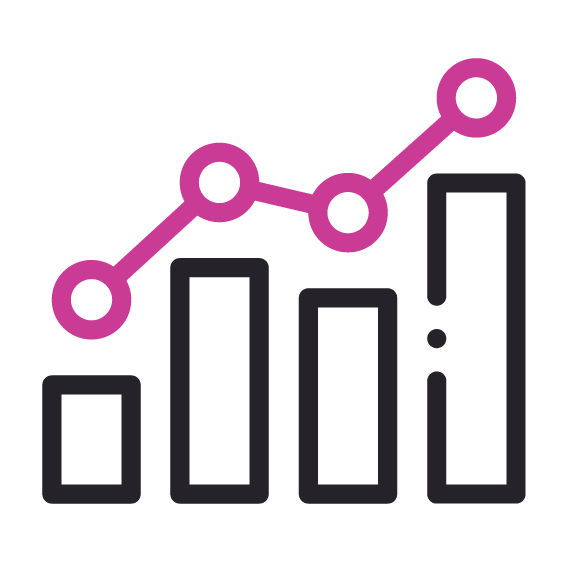
Know the Difference Between Primary and Secondary Metrics
An A/B test may affect multiple KPIs, making it difficult to determine whether the test is a winner. For this reason, it’s best practice to outline the experiment’s primary and secondary metrics to help it stay focused.
- Primary Metric: The test’s primary metric should align with your main KPI and is the determining factor of the experiment’s success.
- Secondary Metrics: Two to five secondary metrics help you gain insights for long-term success. These provide information about a customer’s behavior regarding the changes on the site.
- Monitoring Metric: These metrics don’t determine whether a test is a winner or loser, but they can provide additional information about the experiment.
It’s best practice to outline your experiment’s primary and secondary metrics to help you stay focused.
Let’s apply these terms to our ecommerce company, Bizness Inc., and its Apple Pay checkout experiment.
- Primary Metric: For this A/B test, the primary metric looks at the number of users who complete an order after entering the checkout process to see if implementing Apple Pay would potentially lead to more customers purchasing the product.
- Secondary Metrics: In Bizness’ Apple Pay experiment, the secondary metrics would be average order value and cart abandonment rate. These metrics don’t determine the overall success of the test, but they indicate the potential long-term success of implementing Apple Pay. Decreased cart abandonment and more users placing more orders would show that Apple Pay makes the checkout process more efficient and leads to a higher conversion rate.
- Monitoring Metric: Monitoring metrics include Apple Pay usage rate, payment errors, and page load time. These provide insights into improvement areas and any necessary changes. If Bizness sees more conversions with Apple Pay, or if website users run into more errors or longer page load time, it could indicate whether leaders need to implement changes to improve the user experience.
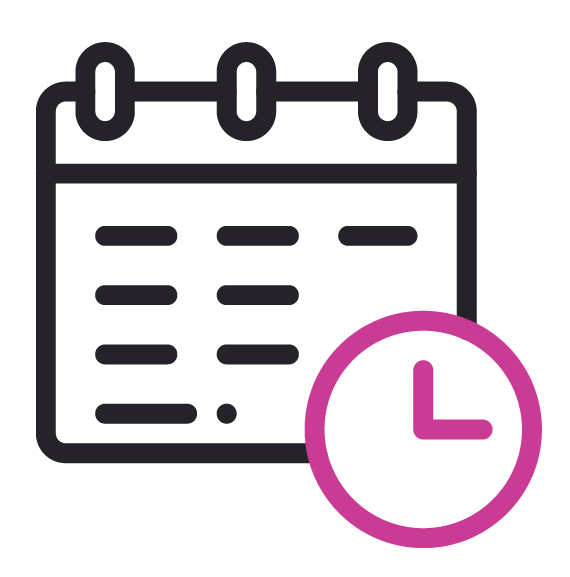
Set up a test timeline of two to eight weeks
Before setting a time frame for your A/B test, consider the number of monthly visits and conversions your website reaches. For example, if your site only gets around 1,000 conversions per month, it may take months for a test to reach statistical significance. Alternatively, reaching the minimal detectable effect would be less costly and time-consuming.
When setting up an experiment:
- Aim for a test duration between two and eight weeks (no longer than eight weeks).
- Lower conversion rates can extend the test duration, increasing costs without guaranteeing positive results.
- Achieving statistically significant results may take longer, with fewer conversion results.
Many platforms exist that can help you determine the appropriate length of time for an A/B test. Some of these tools are even free, such as the Adobe Target Sample Size Calculator and the VWO Sample Size Calculator.
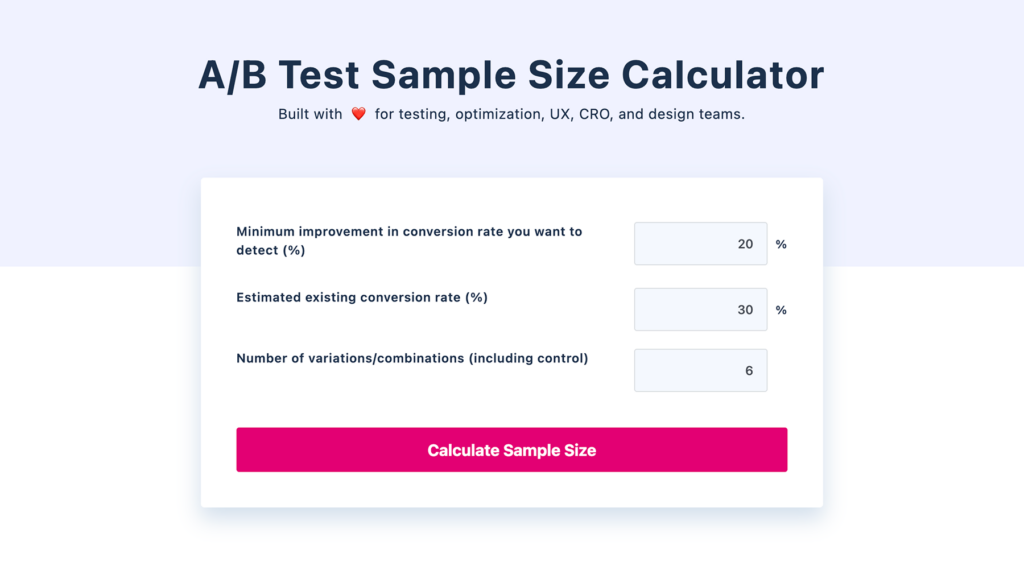
If you’re working in Adobe Analytics, you can pull in metrics from a previous period to assist you in determining how long the test will take and whether the cost would be worth the time. If you don’t want to use Adobe Target, multiple third-party online calculators can get the job done.
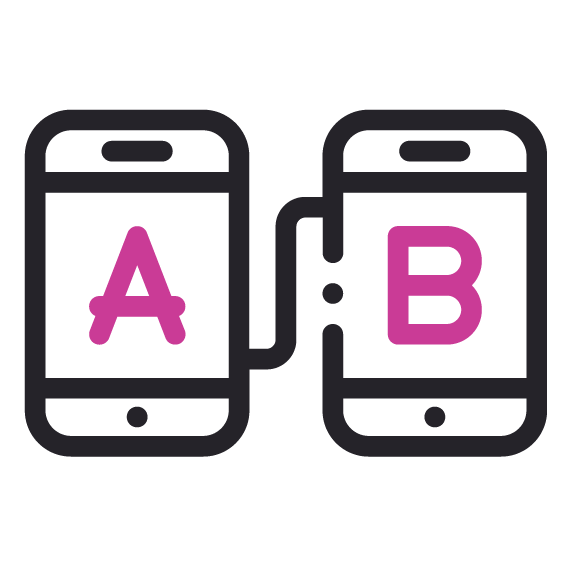
Limit the number of variations in one test
Similarly to the test timeline, the number of experiment variations you run should vary depending on your website’s traffic and conversions. More variations lead to smaller sample sizes. If your site sees a high enough volume of traffic that you can run multiple variations, that’s great! However, if you attempt to run multiple variations without sufficient traffic, it may extend the test duration and complicate the process of reaching statistical significance.
Here’s what to remember:
- Fewer variations means more users per variation, leading to larger sample sizes for both the control and the variant.
- More variations may increase the risk of false positives while extending the time it takes to achieve significant results.
Attempting to run multiple experiment variations without sufficient traffic may extend the test duration and complicate the process of reaching statistical significance.
If you run one variation and a control, your user base will be split 50/50. Adding two more variations would separate the user base into four groups, likely extending your test timeline, making it more difficult to reach statistical significance, and increasing the chances of false positives.
If you want to test multiple test variations, take a more strategic approach. Here are some best practices we recommend:
- Focus on the hypothesis. Look into how each variation strategically relates to your hypothesis and aligns with driving your primary metric.
- Use sequential testing. If you are unsure which variation to run, consider testing each one at a time. If the first variation succeeds, run a second test against that variation.
- Ensure the traffic is there. The biggest challenge with testing multiple variations is the amount of traffic being split up into smaller groups. Ensure there is an adamant amount of traffic to avoid false positives or extending a test too long.
A proactive approach to A/B testing enhances the credibility of your findings and builds trust and alignment among team members.
Following the above best practices will benefit you in the long run when working with your team. Taking these extra steps will enable you to back up your results more effectively and demonstrate the rationale behind your A/B tests. It will also foster better communication and collaboration within your team, ensuring everyone understands the experiment’s objectives and outcomes.
Need more help? BlastX is here to guide you
Taking a proactive approach to A/B testing enhances the credibility of your findings and builds trust and alignment among team members, ultimately driving more successful and data-driven decision-making.
If your organization struggles to extract actionable insights or design experiments aligned with your business goals, BlastX can guide you through the process. Our experimentation/personalization consulting experts can help you design, implement, and refine your A/B testing strategies and roadmap, ensuring you gain the most from your experiments.